AI Governance for Corporates
Learn how to govern AI in an ethical, transparent, and compliant manner. Explore frameworks and key governance practices.
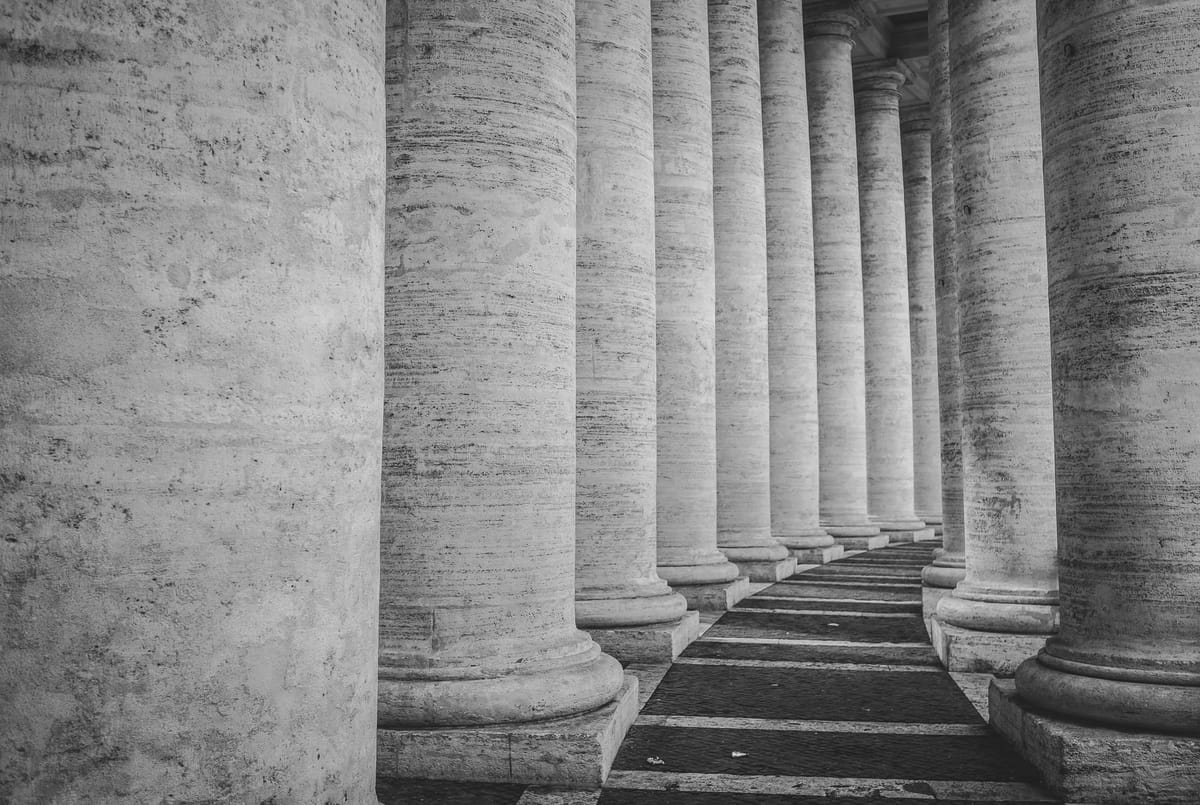
As artificial intelligence (AI) continues to transform industries and redefine business practices, the importance of governing these systems responsibly has never been more critical. AI governance ensures that AI technologies are developed and deployed ethically, transparently, and in a manner that mitigates risks while maximising benefits.
Understanding AI governance
AI governance refers to the policies, procedures, and structures that organisations implement to oversee the development, deployment, and use of AI systems. This governance is crucial for ensuring that AI operates ethically, legally, and in alignment with societal values. Key components of AI governance include:
- Ethical Guidelines: Standards guiding the moral implications of AI applications
- Compliance: Ensuring AI systems adhere to relevant laws and regulations
- Risk management: Identifying and mitigating potential risks associated with AI
- Accountability: Establishing clear responsibilities and oversight mechanisms
Effective AI governance helps organisations avoid biases, ensure transparency, protect privacy, and maintain public trust in AI technologies.
Example of a governance framework: Singapore Model AI Governance Framework
The Singapore Model AI Governance Framework is a leading example of a comprehensive approach to AI governance. Developed by the Infocomm Media Development Authority (IMDA) of Singapore, this framework provides practical guidelines for organisations to deploy AI responsibly.
The Model Framework is based on two high-level guiding principles that promote trust in AI and understanding of the use of AI technologies:
- Organisations using AI in decision-making should ensure that the decision-making process is explainable, transparent, and fair.
- AI solutions should be human-centric. The protection of the interests of human beings, including their well-being and safety, should be the primary considerations in the design, development, and deployment of AI.
The focus area of the framework are:
- Internal Governance Structures and Measures: Defining and communicating clear roles and responsibilities, ensuring the monitoring and management of risks, and training staff.
- Human Involvement in AI-Augmented Decision-Making: Implementing an appropriate degree of human involvement and minimising the risk of harm to individuals.
- Operations Management: Minimising bias in data and models and ensuring explainability, robustness, and regular tuning.
- Stakeholder Interaction and Communication: Engaging with stakeholders, including employees, customers, and regulators, to make AI policies known to users, address concerns, provide the opportunity for feedback, and maintain transparency.
This framework is accompanied by case studies and implementation guides, making it a valuable resource for companies aiming to harness AI while maintaining high standards of governance.
Implementing a governance framework
Implementing a governance framework such as the Singapore Model AI Governance Framework involves several steps.
- Internal governance structures and governance
- Clear roles and responsibilities: Allocate responsibility for and oversight of various stages and activities involved in AI deployment to the appropriate personnel or departments. Ensure everyone involved is fully aware of their roles and responsibilities, properly trained, and provided with the necessary resources and guidance.
- Governance committee: Form a multidisciplinary team responsible for overseeing AI initiatives, including representatives from IT, legal, compliance, ethics, and business units to ensure comprehensive oversight.
- Risk management: Implement a sound system of risk management and internal controls that specifically addresses the risks involved in the deployment of the selected AI model.
- Determining the level of human involvement in AI-augmented decision-making
- Develop a methodology to aid organisations in setting their risk appetite for the use of AI, determining acceptable risks, and identifying an appropriate level of human involvement in AI-augmented decision-making.
- The framework identifies three broad approaches to classify the various degrees of human oversight in the decision-making process:
- Human-in-the-Loop: Human oversight is active and involved, with humans retaining full control and AI only providing recommendations or input.
- Human-out-of-the-Loop: No human oversight over the execution of decisions. The AI system has full control without the option of human override.
- Human-over-the-Loop (or Human-on-the-Loop): Human oversight is involved to the extent that the human is in a monitoring or supervisory role, with the ability to take over control when the AI model encounters unexpected or undesirable events.
The framework also proposes a design framework (structured as a matrix) to help organisations determine the level of human involvement required in AI-augmented decision-making.
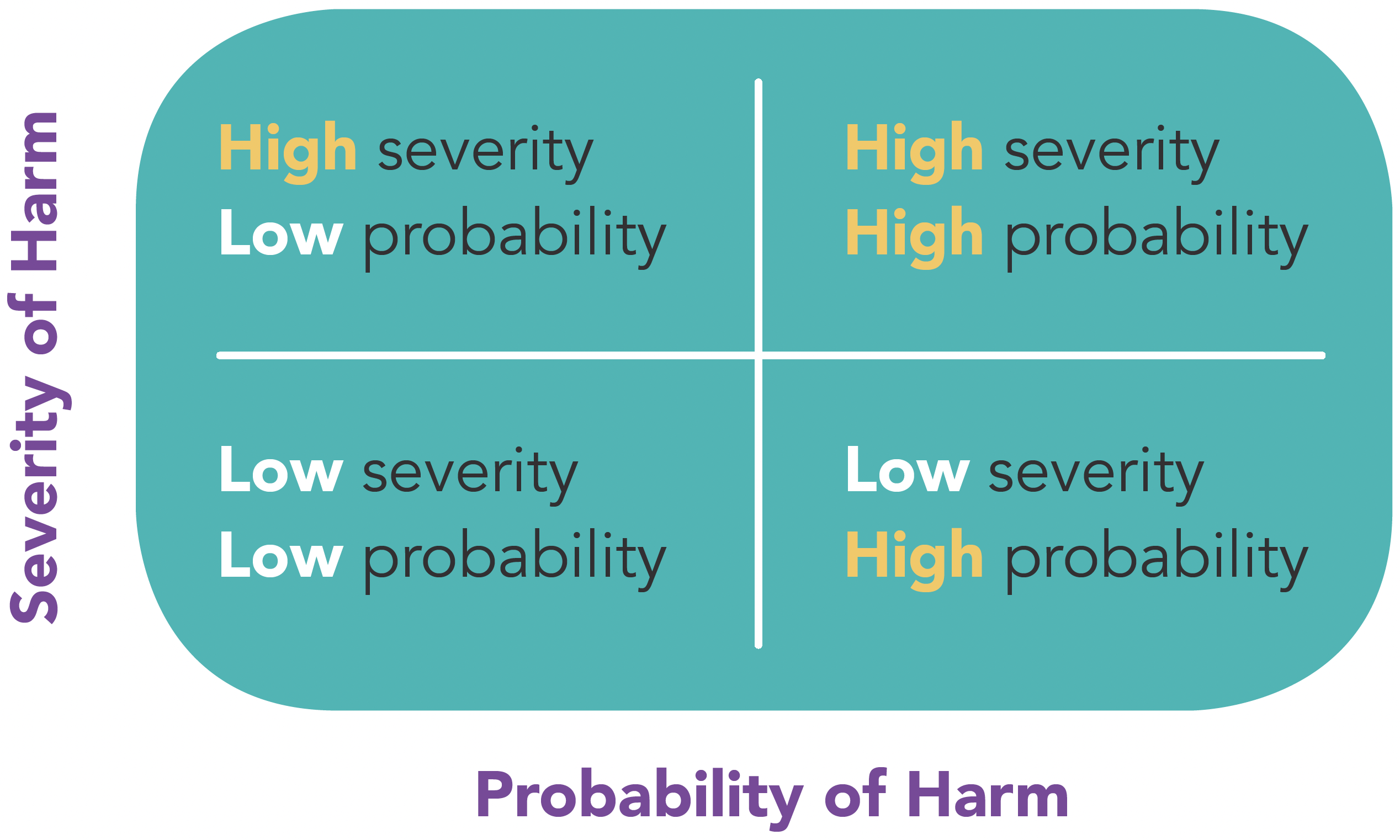
- Operations Management
- Adopt responsible measures in the operations aspect of AI development and deployment, sometimes referred to as MLOps.
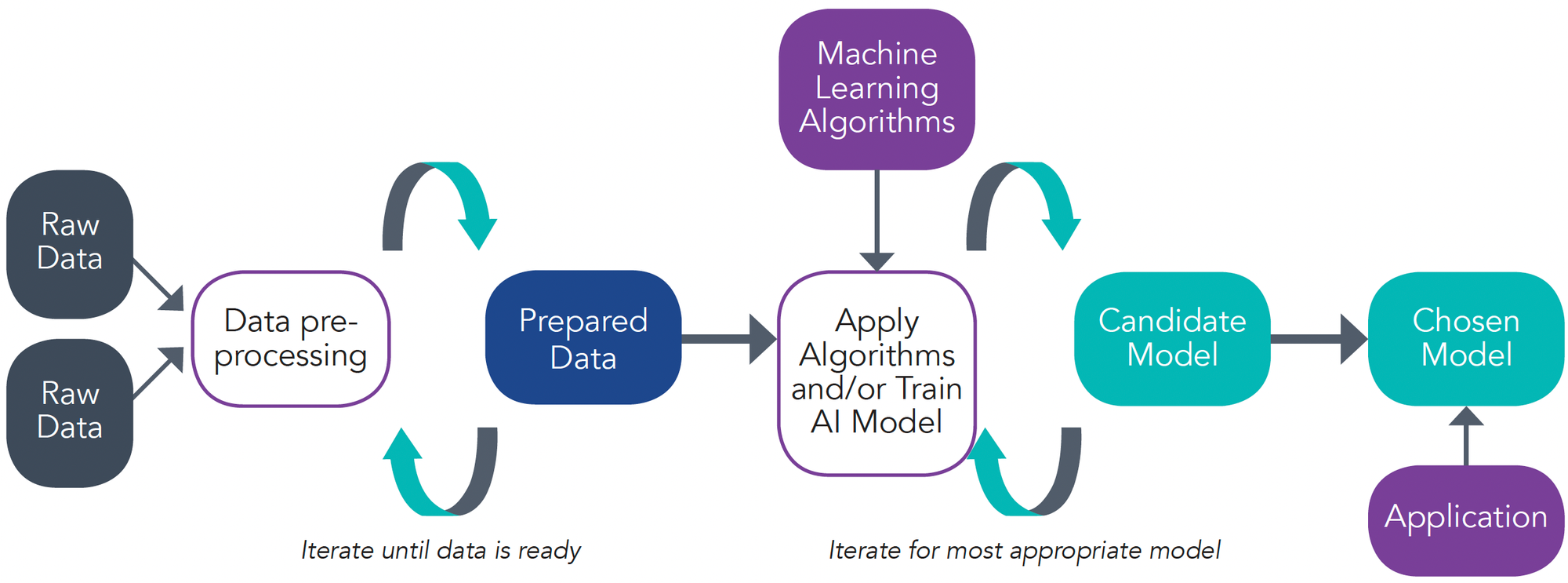
This spans across the following areas:
- Data: Ensure that models are built using unbiased, accurate, and representative data. Relevant departments within the organisation with responsibilities over data quality, model training, and model selection must work together to implement good data accountability practices.
- Explainability: Explain how deployed AI models' algorithms function and how the decision-making process incorporates model predictions to build understanding and trust.
- Repeatability: Document the repeatability of results produced by the AI model to ensure consistency in performance.
- Robustness: Ensure that deployed models can cope with errors and erroneous input to build trust in the AI system.
- Regular tuning: Ensure that deployed models can cope with errors and erroneous input to build trust in the AI system.
- Traceability: Document the AI model's decisions and the datasets and processes that yield the model’s decisions in an easily understandable way.a
- Reproducibility: Ensure that an independent verification team can reproduce the same results using the same AI method based on the organisation's documentation.
- Auditability: Prepare the AI system for assessment of its algorithms, data, and design processes.
- Stakeholder interaction and communication
- Develop strategies for communicating with stakeholders and managing relationships. This includes:
- General disclosure: Provide information on whether AI is used in products or services, how AI is used in decision-making, its benefits, and the steps taken to mitigate risks.
- Policy for explanation: Develop a policy on what explanations to provide to individuals and when to provide them.
- Interacting with consumers: Consider the information needs of consumers as they interact with AI solutions.
- Option to opt-out: Decide whether to provide individuals with the option to opt-out from the use of AI products or services.
- Feedback channels: Establish channels for raising feedback or queries, potentially managed by a Data Protection Officer or Quality Service Manager.
- Testing the user interface: Test user interfaces and address usability problems before deployment.
- Easy-to-understand communications: Communicate in an easy-to-understand manner to increase transparency.
- Acceptable user policies: Set out policies to ensure users do not maliciously introduce input data that manipulates the performance of the solution’s model.
- Interacting with other organisations: Apply relevant methodologies when interacting with AI solution providers or other organisations.
- Ethical evaluation: Evaluate whether AI governance practices align with evolving AI standards and share the outcomes with relevant stakeholders.
By following these steps, organisations can effectively integrate the Singapore Model AI Governance Framework, ensuring that their AI deployments are both innovative and responsible.
Future of AI governance
The future of AI governance is likely to be shaped by several emerging trends and challenges:
- Evolving regulations: As AI technologies advance, regulatory frameworks will need to adapt to address new ethical and legal issues.
- Global collaboration: International cooperation will be essential for developing consistent and effective AI governance standards.
- Technological advancements: Continuous innovation in AI will require governance frameworks to be flexible and adaptive.
- Public awareness and trust: Building and maintaining public trust in AI will be crucial, necessitating transparent and accountable governance practices.
The dynamic nature of AI development means that governance frameworks must evolve continuously to address new challenges and opportunities.
Additional Resources
For further reading and to explore more frameworks on AI governance, consider the following resources:
- OECD AI Principles: The OECD AI Principles are the first intergovernmental standards on AI, promoting AI that is innovative, trustworthy, and respects human rights and democratic values.
- Ethics Guidelines for Trustworthy AI by the European Commission: These guidelines outline key requirements for AI systems to be trustworthy, including human agency and oversight, technical robustness, and privacy and data governance.
- IBM's AI Fairness 360: AI Fairness 360 is an open-source toolkit developed by IBM to help detect and mitigate bias in AI models throughout the AI lifecycle.
- Google AI Principles: Google’s AI Principles aim to guide the ethical development and use of AI technologies, emphasising socially beneficial applications and the need to avoid creating or reinforcing unfair biases.